Newsroom
Computational modelling breakthrough from the Zandstra lab reveals new insights into the potential emergence and treatment of disease
A breakthrough paper from the Zandstra lab has scientists excited about the potential impacts of their findings on personalized medicine. The research in the Stem Cell Reports publication showcases a new computational modelling technique that helps understand the relationship between genes, cells and their microenvironments (molecules, cells and structures that surround and support other cells and tissues). The team designed computer models to make predictions about cell fates and development and confirmed those predictions with in vitro stem cell experiments. The first model of its kind to take into account multi-scale interactions (interactions from the level of genes, to cells and up to tissues), lead author Dr. Himanshu Kaul (he/him) describes the impact of these findings on personalized medicine and stem cell therapies.
“The ability to study multi-scale interactions to predict how a certain tissue might grow or certain disease emerge is quite powerful. We can predictably control the fate of certain cells by developing models like this. This tool helps us understand the molecular logic underpinning biological processes, enabling us to design cells on demand. This approach also empowers us to start understanding diseases at the patient specific level,” explains Kaul.
Dr. Kaul, a former postdoctoral fellow from the Zandstra lab, is now a Royal Academy of Engineering Research Fellow running his own lab at the University of Leiscester. Kaul has long been trying to understand gene regulatory networks (GRN -networks of genes that influence and regulate each other’s behaviour) and combine that knowledge with clinical data. He received his PhD from the University of Oxford where he developed software frameworks to help understand how cells impact their microenvironment and are in turn impacted as a result of those changes. Attracted to the Zandstra lab’s expertise working with GRNs Dr. Kaul joined them in 2016.
“I was developing virtual asthma patient models and was able to make predictions that were consistent with the clinical data we were seeing. But when I tried to make patient specific predictions, I saw some of the numbers were way off and I realized I was missing something. That was Gene Regulatory Networks. So, I wanted to join the Zandstra lab to learn how to incorporate GRNs into my model and also develop experimental skills,” recounts Kaul.
Understanding the multi-scale interactions that shape cell fate sets the stage for programming specific multicellular outcomes on demand. Scientists designing cell therapies will be able to leverage these computational models to virtually ‘tweak their recipes’ before embarking on physical experiments and scaling efforts, saving years of time in the lab and cutting costs significantly. Subsequently, the approach can be used to create virtual patient profiles (digital representations of a patients’ physiology); a powerful tool for personalized medicine research. One application area for these techniques in Dr. Kaul’s new lab is asthma research.
“If I want to study how asthma is emerging, this framework will allow us to understand how the interactions of certain genes are important and lead to changes at the cellular level, and in turn how that leads to the structural changes in the airways of a specific patient,” Kaul explains.
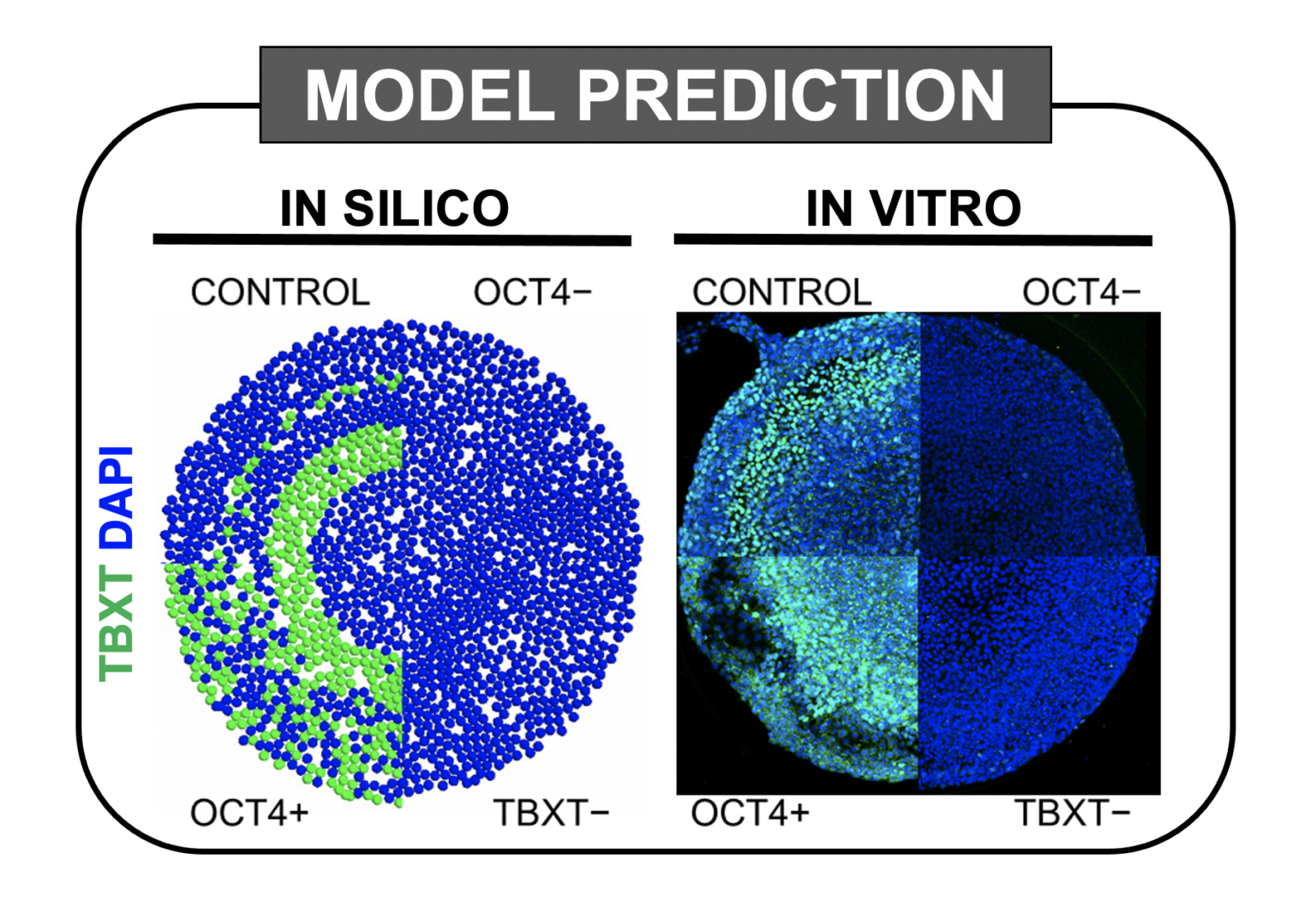
Computer model accurately predicted (left) how different perturbations will impact certain cells (labelled green) in vitro (right).
A better understanding of multiscale interactions could lead to advancements in personalized medicine.
“Now we are trying to use this approach to understand why some patients would respond to a certain therapy over others, allowing us to tailor our treatment to individual patients,” says Kaul.
In the early stages of their application, these techniques show broad potential. In addition to tailoring patient therapies, they can also be used to improve the manufacturing of cell types in bioengineering and create a better understanding of how diseases may emerge through multiple genes using GRNs in virtual cells. Dr. Kaul reflects on the success of these new computational models:
“All mathematical models are imperfect, but some are useful. There are always assumptions that you make. Whether those assumptions stand or not comes out during validation. The fact our validation has been quite robust gives me great confidence that what we’ve captured is biologically significant.”
The findings from this research will help scientists better understand how diseases may emerge through multiple genes, how to develop effective personalized therapies and efficiently bioengineer cells in the laboratory. Dr. Kaul and his collaborators look towards further developments of this research with much hope and excitement for what’s to come.
Quick links:
- Read the publication: Virtual cells in a virtual microenvironment recapitulate early development-like patterns in human pluripotent stem cell colonies
- Learn more about this research and listen to the podcast episode Computing Positional Cues: From Single Cells to Embryo Development on The Stem Cell Report with Martin Pera
- Read about and download the GARMEN: GRN Agent-based Reaction-Diffusion Modeling Environment computer model
- Learn more about Dr. Himanshu Kaul and the Kaul lab: https://www.kaullab.com/
- Learn more about Dr. Peter Zandstra and the Zandstra lab